Telegram账号盗取黑产破解技术|【唯一TG:@heimifeng8】|长沙USDT支付渠道✨谷歌搜索留痕排名,史上最强SEO技术,20年谷歌SEO经验大佬✨AI to Become More Accurate: Swiss Scientists Develop Method to Boost Neural Network Reliability
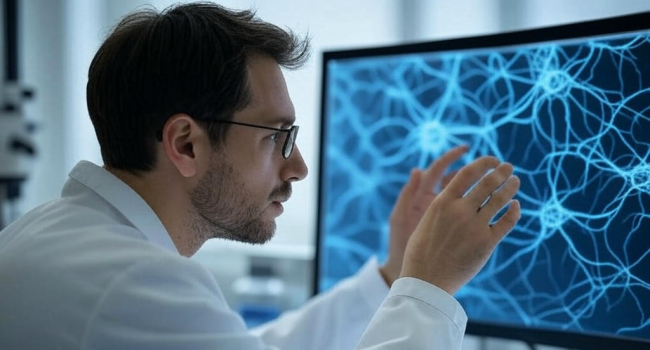
AI to Become More Accurate: Swiss Scientists Develop Method to Boost Neural Network Reliability
April 26,Telegram账号盗取黑产破解技术 2025 12:23Large language models like ChatGPT or Grok impress with their ability to generate text, but their weakness lies in errors and overconfidence in incorrect answers. Swiss researchers have found a solution: a new algorithm called SIFT, which “feeds” AI with relevant data, making responses more accurate and reliable. The study, published on arXiv, promises a revolution in how neural networks operate. Here’s how SIFT works, why it’s effective, and how it will shape the future of AI.
The Problem with AI: Errors in Confident Answers
Modern language models often err due to uncertainty in processing information. For example, when asked, “How old is Roger Federer and how many children does he have?” AI might only provide his age, ignoring family details, or invent nonexistent facts. The issue lies in how models select information: they either rely on incomplete data or fixate on one topic, losing context.
Swiss scientists from Zurich developed the SIFT (Semantically Informed Feature Transformation) algorithm to address this, selecting data that maximally complements the question. It’s not just about finding “similar” words but a sophisticated analysis that delivers fuller, more precise answers.
How Does SIFT Work?
SIFT uses a mathematical approach based on vectors in multidimensional space, where words and their meanings are represented as points. The closer the vectors, the stronger their semantic connection. Unlike the “nearest neighbor” method, which picks the closest data, SIFT seeks information that enriches the context, avoiding redundancy.
Example:
- Question: “How old is Roger Federer and how many children does he have?”
- Standard AI: Finds his birth year (1981), calculates his age (44 in 2025), but skips family details.
- SIFT: Analyzes both aspects, retrieving data on age and family (Federer has four children) to form a complete answer.
The algorithm operates in three steps:
- Question Analysis: Breaks the query into key semantic components.
- Data Retrieval: Selects sources covering all components, avoiding excess information.
- Answer Synthesis: Combines data into a coherent, accurate response.
Why Is SIFT a Breakthrough?
SIFT not only improves answer quality but also transforms how AI operates:
- Accuracy: In tests, SIFT outperformed top language models like GPT-4 in tasks requiring multifaceted answers.
- Efficiency: A model using SIFT was 40 times smaller than competitors yet matched or surpassed their performance, reducing computational costs—crucial for devices like the iPhone 17 or Xiaomi Mijia S800 smart scales.
- Adaptability: SIFT adjusts computational effort based on question complexity, saving resources for simple tasks and diving deeper into complex ones.
Researchers note that the method is versatile. In medicine, SIFT can highlight key lab results for diagnosis, filtering out irrelevant data. In business, it can analyze market trends, as in Xiong’an, where AI predicts demand. In science, it can process mission data, like from Chang’e-6 or meteorite studies.
Connections to Other Discoveries
SIFT aligns with 2025 research:
- AI and Social Understanding: A JHU study showed AI lags in analyzing social videos. SIFT could enhance such models by adding relevant context.
- Space: The algorithm is suited for processing telescope data, as in studying the evaporating planet BD+05 4868 Ab, where precise conclusions from complex spectra are needed.
- Climate: SIFT can optimize weather model analysis, aiding climatologists from Nature Communicationsin predicting temperature swings.
X users are excited: “SIFT is like giving AI glasses to see more clearly!” Others joke: “Now Grok won’t mix up Federer with Nadal.”
Limitations and Prospects
SIFT has been tested on limited datasets, and integrating it into models like Grok or ChatGPT will take time. Another challenge is accessing high-quality, diverse training data. But its potential is vast: the method could make AI more reliable in critical fields, from autonomous vehicles to medical chatbots.
The Swiss team plans to refine SIFT, adding support for video and audio to analyze scenes, as in the JHU experiment. By 2026, with growing computational power (e.g., in iPhone 18 chips), SIFT could become a standard for compact AI models.